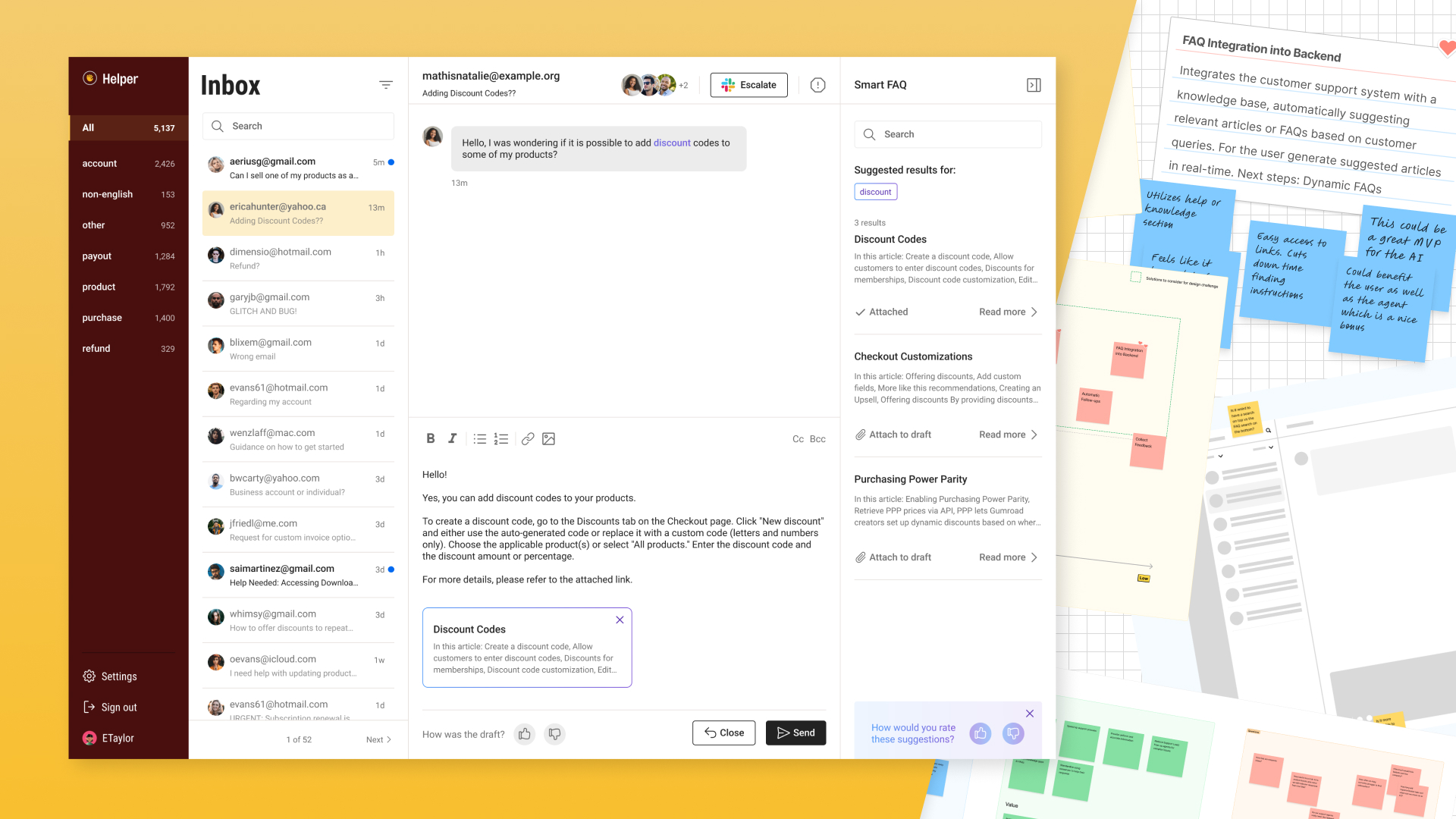
Streamlining Customer Support with AI-powered Smart FAQs
Project
Gumroad | AI-Powered Customer Support
Role
Product Designer | Research, design thinking, rapid prototyping, end-to-end design, visual coding
Team
My co-designer, Pania and me
Tools
Figma, Figjam, Rotato, Helper.ai, ChatGPT, Cursor
Summary
To demonstrate our design and problem-solving capabilities, Pania and I created an AI-powered Smart FAQ system for Gumroad’s Helper.ai platform. This solution aimed to automate repetitive tasks, speed up response times, and provide uniform, accurate information, freeing up support agents for more complex issues. Our project showed how design thinking and rapid prototyping can tackle real-world challenges within a tight timeframe.
Hours
Designers
Lines of Visual Code
Awesome Solution
The Problem
Gumroad’s support team struggled with inefficiencies caused by repetitive questions and underutilized knowledge bases, leading to slower response times and inconsistent answers. The need was clear: automate mundane tasks, improve response consistency, and enable agents to handle more complex inquiries.
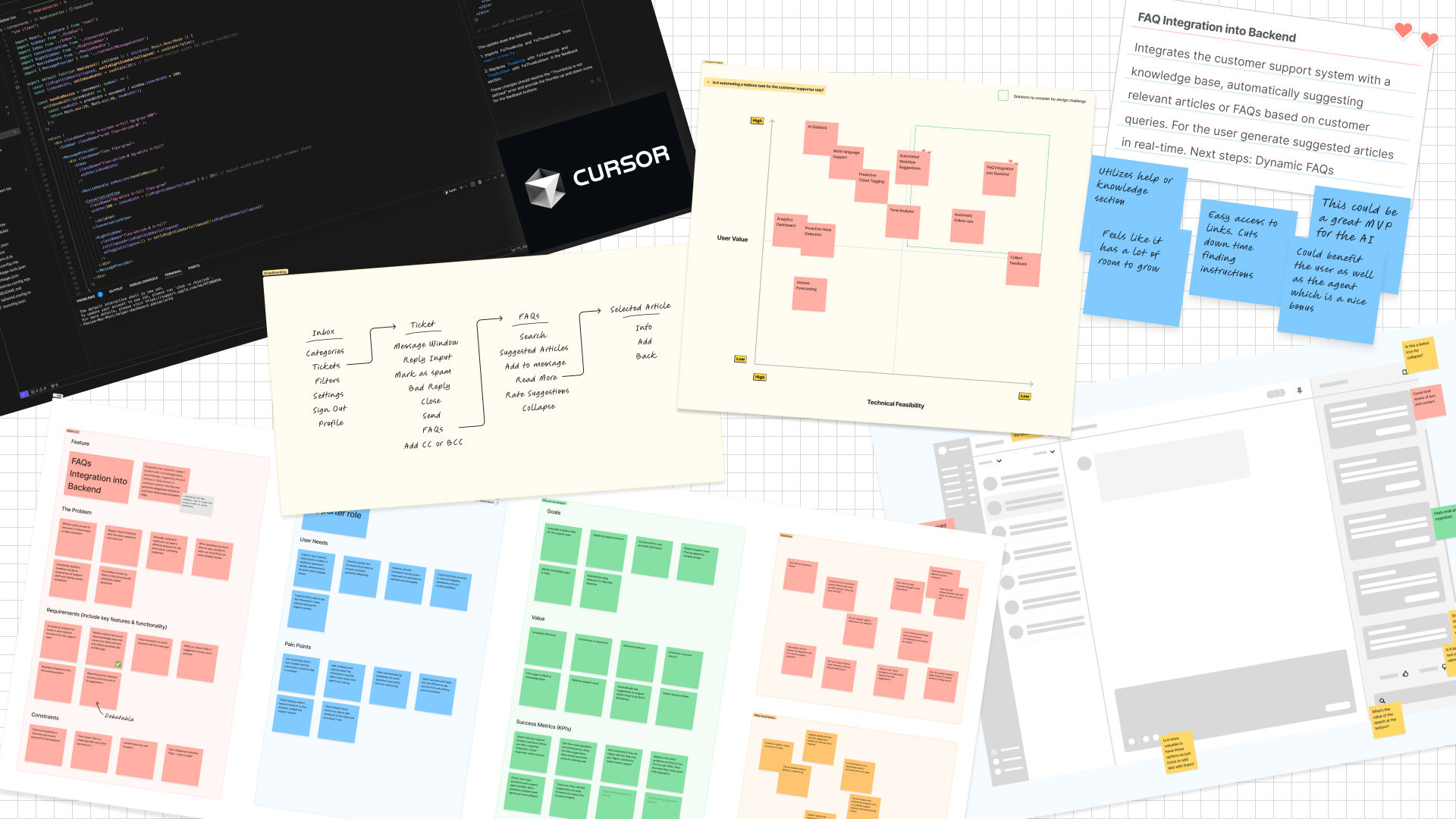
Strategy
We combined rapid ideation with iterative design to develop a user-centric solution. Starting with a brainstorming session, we mapped out high-impact features, created a product requirement document (PRD), and moved through breadboarding, wireframing, and high-fidelity prototyping. Our process also included visual coding using Cursor to generate the functional design code.
Goals
- Automate repetitive support tasks.
- Speed up customer response times.
- Provide consistent and accurate information to customers.
- Reduce support load, freeing agents for complex issues.
- Identify gaps in FAQs and optimize knowledge base usage.
Constraints
- Tight weekend-long timeline for the design challenge.
- Lack of real user data to validate initial assumptions.
- Limited resources for in-depth research or implementation.
Target Audience
The intended users for this project are Gumroad’s customer support agents and the customers they serve. Support agents need tools that streamline their workflow, allowing them to respond to customer inquiries quickly and accurately, while customers expect fast, reliable answers to their questions.
Pain Points
- Support agents spend too much time answering repetitive questions.
- Inconsistent responses due to underutilized knowledge bases.
- Delays in resolving complex inquiries as agents handle mundane tasks.
- Customers experience slower response times and inconsistent information
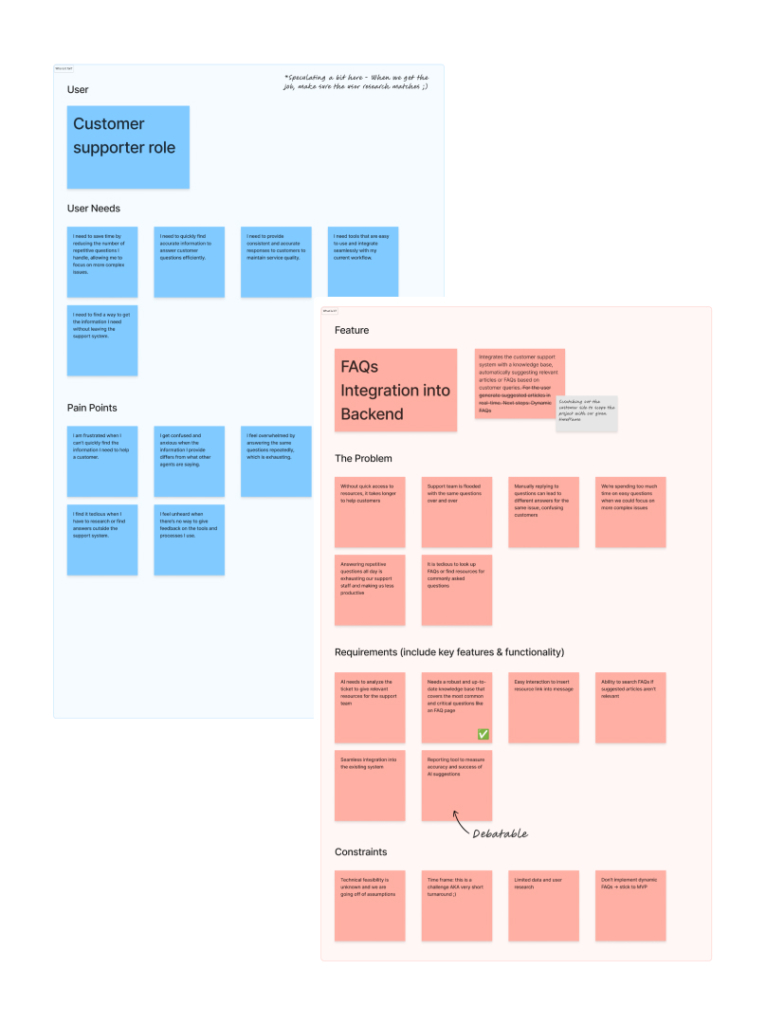
Solution
We developed an AI-powered Smart FAQ system integrated into the Helper.ai interface. This solution automatically suggests relevant knowledge base articles, streamlines agent workflows, and allows for one-click insertion of AI-generated responses. A feedback loop was built into the system for continuous improvements to suggestions.
Here’s how we tackled the challenge:
- Conducted rapid brainstorming and prioritization sessions.
- Created a comprehensive product requirement document (PRD).
- Sketched and wireframed possible solutions.
- Developed high-fidelity prototypes using Figma.
- Generated front-end code using Cursor for visual coding.
- Produced branding videos to enhance the presentation.
Results & Impact
Though we couldn’t measure the impact in real-time due to the nature of the design challenge, our solution has the potential to:
- Significantly reduce response times.
- Increase first-contact resolution rates.
- Improve customer satisfaction by providing quick and accurate information.
- Enable agents to focus on complex issues, leading to better overall support performance.
What's Next...
The next logical step would be conducting user research to validate assumptions and make refinements based on actual user feedback. Additionally, creating a detailed roadmap for scaling the feature and ensuring its technical feasibility is essential.
/Fin
This design challenge demonstrated our ability to rapidly conceptualize, design, and prototype complex features while balancing user needs with business goals. Working under tight time constraints taught us to make quick yet thoughtful decisions and emphasized the importance of early assumption validation. Collaboration highlighted the value of diverse perspectives in solving problems, and we learned to consider technical feasibility earlier, particularly when integrating AI. Additionally, the project brought to light the ethical implications of AI, reinforcing the need to prioritize ethical design from the outset.